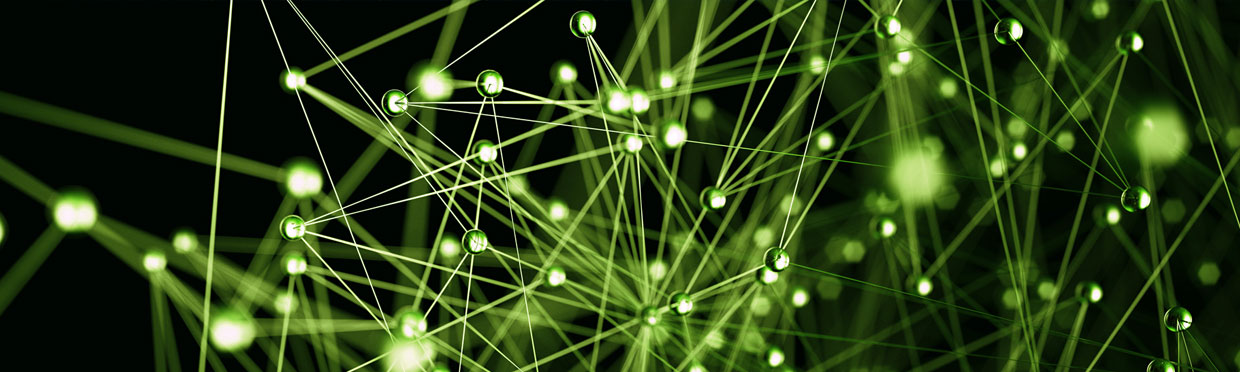
The knowledge network is the brain of precision medicine, with the informatics power to aggregate all types of biological information into an information commons, stratify it into “layers” of distinct data types, and then discern patterns and connections within and between layers. This process builds a network of knowledge from across disciplines. This new knowledge, in turn, can be visualized and made accessible to researchers and health practitioners.
The knowledge network will pull data out of silos, connecting the wealth of information that already exists from basic molecular research, clinical insights, environmental data and others. The connections and patterns that emerge will suggest testable hypotheses and new conceptual syntheses for researchers, implicate mechanisms of disease for researchers and clinicians, and enable more precise diagnoses and treatments for individual patients. And it will continuously acquire new data – from laboratory experiments and clinical trials to electronic health records and pedometer readings—that will inform our collective understanding of health.
As the network broadens and deepens, a clinician sitting with a patient could access information to help make a tailored assessment, drawing from molecular and demographic datasets, accessing results from patients participating in a recent and related study, connecting that with clinical imaging and behavioral information, and comparing the patient across a population of other patients who are both similar and different. Importantly, building the network is a vast and continuous undertaking, but it need not be complete to contribute in powerful ways. Thus, pilot projects, even on a small scale, can have an impact.
The knowledge network also will enable researchers to interact to share new findings, processes and ideas. Those developing the pilot project are carefully considering provenance: a thorough auditing system will track uploads, downloads and further uses of current data. In addition, the efforts of building the UCSF knowledge network are yielding modular computational tools that can be adapted to a variety of needs and data environments, with an eye to future use by researchers and clinicians with a wide range of needs.
Driving Projects
Developed and led by a comprehensive group of researchers, faculty and staff, The Information Commons is a fast, shared repository of UCSF clinical data, clinical notes, related basic science and population data, and supporting tools on Spark, a next generation Apache-based open-source platform developed at UC Berkeley.
Neighborhood Explorer allows searching SPOKE for a node of interest, such as a specific drug compound, gene, or protein, and seeing what other nodes are in its immediate connectivity neighborhood, such as related diseases, side effects, pathways, and other compounds, genes, or proteins. The search can be limited by filtering to specific node and edge types, and by edge value (for example, compound-treats-disease edges at least clinical trial phase 3 or FDA-approved).
SPOKE (Scalable Precision Medicine Oriented Knowledge Engine) demonstrates the greater Knowledge Network that is at the core of UCSF Precision Medicine. SPOKE offers a graph-theoretic database that will allow researchers to explore these interconnected pathways, enabling new discoveries. SPOKE pulls data out of silos, connecting the wealth of information that already exists from basic molecular research, clinical insights, environmental data and others. It mirrors the very nature of biomedical and health pathways, with millions of entity types including gene, protein, organ, disease condition, drug compounds and side effects – built up from dozens of reference repositories as well as from UCSF clinical evidence.
Wynton is a large, shared high-performance compute (HPC) cluster underlying UCSF’s Research Computing Capability. Funded and administered cooperatively by UCSF campus IT and key research groups, it is available to all UCSF researchers, and consists of different profiles suited to various biomedical and health science computing needs. Researchers can participate using the “co-op” model of resource contribution and sharing.