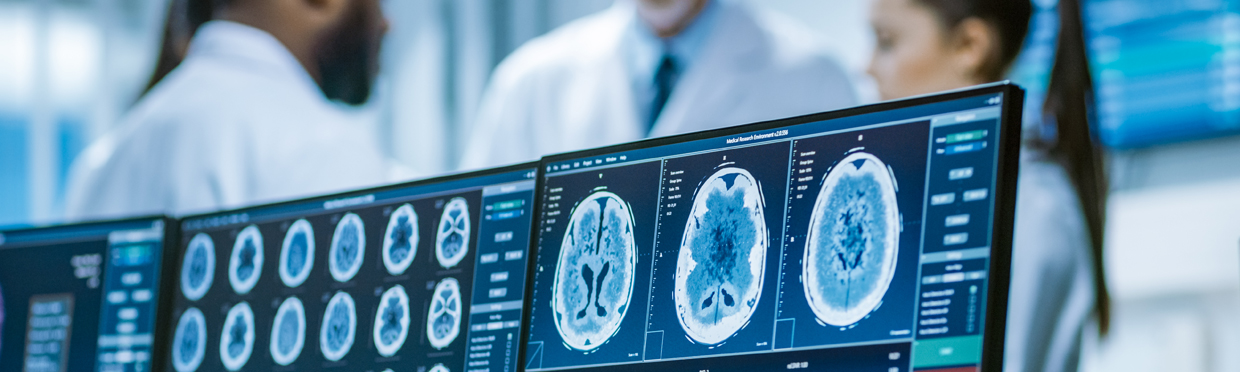
From cryo-electron microscopy, to STORM microscopy, to x-ray crystallography: Novel technologies that were developed in basic science now serve as a bridge to new insights and therapies in the clinic. Experts at UCSF are developing and using the latest imaging tools to enhance diagnosis, improve disease monitoring, and optimize treatment for individual patients. Our scientists apply artificial intelligence in the form of deep learning models to dramatically improve the examination of each patient’s unique medical images, resulting in reduction in time to diagnosis, better accuracy, and improved outcomes.
Leading Centers and Initiatives
- Center for Intelligent Imaging (Ci2):aims to develop strategies that leverage computational tools to improve image acquisition, workflow efficiency, quantitative imaging, and automated diagnosis.
- Center for Imaging of Neurodegenerative Diseases (CIND): is a research center dedicated to studying the causes and effects of neurodegenerative and psychiatric disorders, using imaging techniques such as MRI and PET.
- Department of Radiology and Medical Imaging: a leading health sciences center focused on serving patients, conducting research, and training the next generation of radiologists.
- Department of Ophthalmology: The Department of Ophthalmology and the Francis I. Proctor Foundation for Research in Ophthalmology are dedicated to understanding the causes and mechanisms of eye diseases, with the goals of preventing, slowing, and restoring sight.
Driving Projects
Sharmila Majumdar, PhD, is leading a new technology development grant from the NIH, as part of the HEAL Initiative and BACPAC consortium, aimed at improving treatments for chronic pain and curbing the rates of opioid use. Dr. Majumdar and a highly-experienced multidisciplinary research team – including UCSF’s Valentina Pedoia, PhD, Cynthia Chin, MD, Duygu Tosun, PhD, Irina Strigo, PhD, and Harvard’s Mary Bouxsein, PhD – bring together extensive expertise in MR bioengineering, advanced MRI data analysis, radiology, neuroscience, neurosurgery, orthopedic surgery, and multi-dimensional analytics to respond to the critical need for clarity in the etiologies of chronic back pain and other disorders of the spine.
Jae Ho Sohn, MD, has combined Deep Learning techniques with brain imaging to discover changes in brain metabolism predictive of Alzheimer’s disease (AD). Previous research had demonstrated a link between patterns of glucose uptake in the brain and the disease process, but biomarker discovery was lacking. The nteam trained a DL algorithm using more than 2,100 18-F-fluorodeoxyglucose PET scans from 1,002 patients – a dataset originating from the Alzheimer’s Disease Neuroimaging Initiative. After testing on an independent set of 40 scans from 40 patients never studied, the algorithm was able to predict every case that advanced to AD. Dr. Sohn’s next steps could include a larger multi-institutional prospective study using the algorithm, as well as training the tool further to spot patterns associated with the accumulation of beta-amyloid and tau proteins – a known AD biomarker.
Andreas Rauschecker, MD, PhD, is developing an AI system for probabilistic brain MRI diagnoses. This system computationally models a neuroradiologist’s process of image interpretation by using a convolutional neural network for detection of imaging abnormalities, image processing for quantitative descriptions of these abnormalities in terms of signal, location, and volumetric features, and a probabilistic integration of these derived features with clinical features in the form of Bayesian inference, ultimately arriving at a probability-ranked differential diagnosis. This set of methods has been applied to 50 unique neurological diseases, where the AI system has performed with promising results.
An inter-disciplinary team led by Valentina Pedoia, PhD, including Sharmila Majumdar, PhD, Benjamin Glicksberg, PhD, and Atul Butte, MD, PhD, is developing a multi-modal framework integrating genomics, imaging, and clinical data to develop novel graph-based deep learning predictive models enabling the extraction of latent multi-domain signatures of unique clinical progression trajectories and prognose subject’s future incidence of joint pain. While integrating genomics with imaging and clinical information will shed light on the contribution of heritability in joint pain and contribute to the discovery of novel structural and functional joint imaging pain biomarkers, inconsistencies between joint abnormalities and patient experience may still be observed.